​
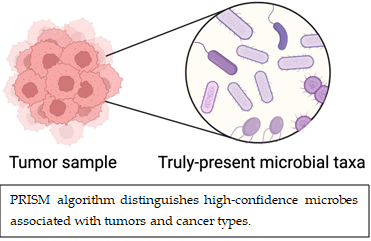
Invention Summary:
The human microbiome plays a critical role in health and disease, influencing conditions such as cardiovascular disease, cancer, and autoimmune disorders. However, analysis of microbial genetics data has struggled to overcome the effects of false positive and false negative data. Computational methods are further constrained by inadequate benchmarking and the complexity of large datasets. To address these issues, improved experimental protocols and more robust analytical frameworks are needed to reliably identify tissue-resident microbial signals in human genomic data.
Rutgers researchers have developed Precise Identification of Species of Microbiome (PRISM), a computationally efficient method for decontamination and precise identification of microorganisms in genomic sequencing data. The purpose of PRISM is to identify taxa that are truly-present in the source sample and that are not falsely positive artifacts and contaminants. False-positive artifacts are taxa reported by taxonomic classifiers, but which are incorrectly classified; the reads assigned to these taxa did not originate from the reported taxa. Contaminant taxa are correctly identified, but these reads were not present in the source tissue; they were introduced during sample handling. PRISM thus has two key conceptual steps: (1) identifying correctly classified taxa and eliminating false positive artifacts, and then (2) distinguishing between truly-present vs. contaminating taxa. PRISM can facilitate microbiome analysis with a broad zone of confidence, enabling identification of microorganisms with significant molecular and clinical implications.
Market Applications:
• Research tool to accurately identify biomarkers within the microbiome for a target disease / illness.
Advantages:
Intellectual Property & Development Status: Provisional application filed. Patent pending. Available for licensing and/or research collaboration. For any business development and other collaborative partnerships, contact: marketingbd@research.rutgers.edu