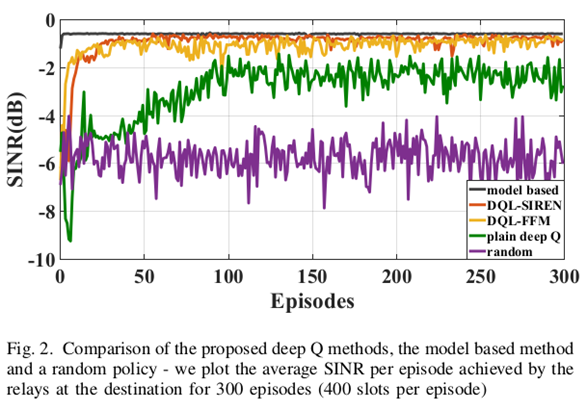
Invention Summary:
Optimal positioning of mobile relays is critical to relay motion control in mobile relay beamforming networks. It requires Channel State Information (CSI) of the potential positions to predict the channel state, which is hard to obtain in a spatiotemporally variable channel.
Rutgers researchers have developed novel deep learning method of guiding relay motion to maximize the cumulative Signal‐to‐Interference+Noise Ratio (SINR) at the destination, where they approached with a model-free deep Q learning method. They proposed two modified Multilayer Perceptron Neural Networks (MLPs) to approximate value function Q. The modifications enable MLP to better learn the high frequency value function and have a profound effect on convergence speed and SINR performance. The two input transformations provide significant performance improvement.
This invention can be a beneficial solution to line-of-sight issues with mmWave technology.
Advantages:
- Higher SNIR
- Reaches maximum SNIR faster than other methods
- DQL methods provide more adaptable and robust solution
Market Applications:
- Disaster Relief
- Search & Rescue
- Aerial Mapping
- Resilient Battlefield Networks
- Autonomous Vehicle Coordination in rural areas
- Cell Network Boost
Intellectual Property & Development Status: Patent pending. Available for licensing and/or research collaboration.
Publication: Evmorfos et al., IEEE Transactions on Signal Processing, 2022. Reinforcement Learning for Motion Policies in Mobile Relaying Networks.