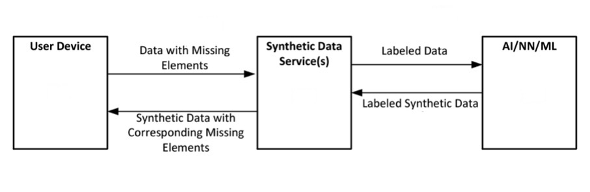
Model illustrating synthetic data generation

Invention Summary:
Developers and analysts often require large, accurately labeled datasets when training AI models, though it can be unrealistically time-consuming and expensive, and in many cases impossible due to privacy and confidentiality, to obtain real-world datasets. Algorithms are developed to generate synthetic data, which instead of the real-world data, are used for modeling and training purposes. However, generating synthetic data that accurately represents original data and preserves missing data has been a challenge. This is despite the fact that missing data can hold crucial information about the problem one is trying to solve.
Rutgers researchers have developed a unique model that enables generating very high-quality and privacy-protecting synthetic data from real datasets while preserving the observable data and missing data distributions. The synthetic data protects privacy as it is generated by a model and not directly collected from any individual. As missing data can often imply significant information that should be included, this invention uses artificial intelligence methods that accurately include missing data values while generating synthetic data. Additionally, incorporating missing data in learning models can help alleviate algorithmic fairness-related problems. Extensive empirical and analytical validation shows that missing data friendly synthetic data generation methods are superior to the current model of discarding or imputing missing data.
Advantages:
This technology:
- Provides high-quality and privacy-protecting synthetic data.
- Includes the ability to retain missing data fields as they were in their original form.
- Formalizes the problem of preserving observable and missing data distribution in synthetic data generation.
Market Applications:
- Healthcare
- Financial Services
- Cyber Security
- Automotive and Robotics
Intellectual Property & Development Status: US Patent filed- 63/405,687. Available for licensing and/or search collaboration.