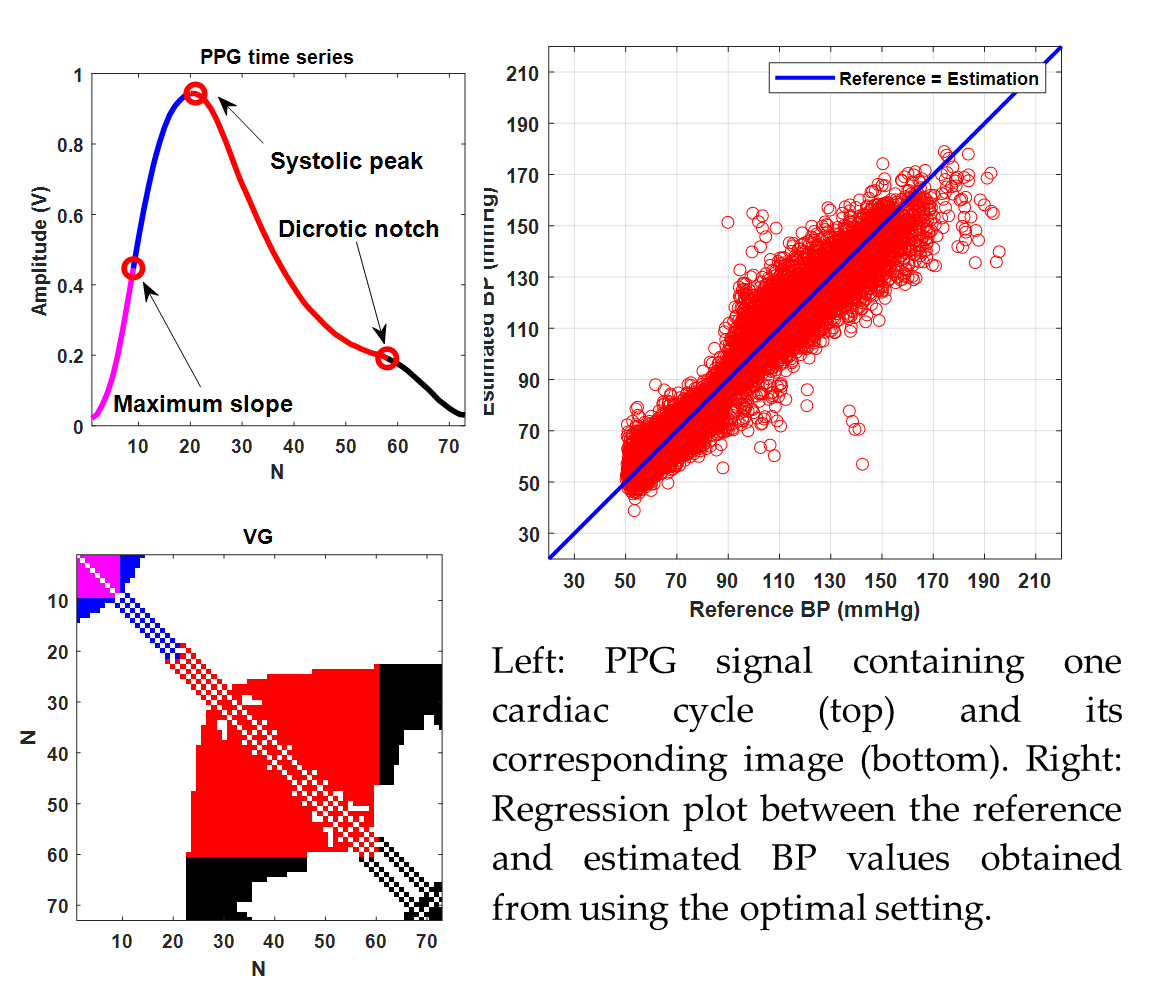
Invention Summary:
Hypertension or high blood pressure affects a large percentage of the global adult population. Frequent self-monitoring of blood pressure (BP) is important for long-term adherence and compliance to clinical advice. However, given the intrinsic inconvenience of conventional cuff- or tonometry-based blood pressure monitors, less than 30% of patients with a BP monitoring device at home measure their BP more than twice a day. There is hence a need for a more convenient, cuff-less alternative, which enables automatic and distraction-free BP monitoring.
Researchers at Rutgers University developed a novel data-driven method for rapid and user-friendly cuff-less photoplethysmogram (PPG)-based BP estimation. This is the first method that proposes to create images from PPG signals via visibility graph (VG) for the purpose of estimating BP via transfer learning from image classification networks. Once the lightweight transfer learning model is trained, it gives estimates of BP from PPG input of duration less than 2 sec.
Using the University of California Irvine (UCI) database consisting of 348 records, the proposed method achieves a best error performance of 0.00 ± 8.46 mmHg for systolic blood pressure (SBP), and 0.04 ± 5.36 mmHg for diastolic blood pressure (DBP).
Advantages:
- Low computation budget
- Computationally fast: ≤ 2 sec raw PPG signal and <50 msec computation time once the model is trained
- Only PPG as input
Market Applications:
- Non-invasive continuous BP monitoring for patients with:
- Hypertension
- Cardiovascular disease
- Spinal cord injury (SCI)
- Home BP monitoring
- Smart watches
- Fitness Trackers
Intellectual Property & Development Status: Patent pending. Available for licensing and/or research collaboration.
Publication: DOI 10.1109/JBHI.2021.3128383, IEEE Journal of Biomedical and Health Informatics.